Wilcoxon签名的测试
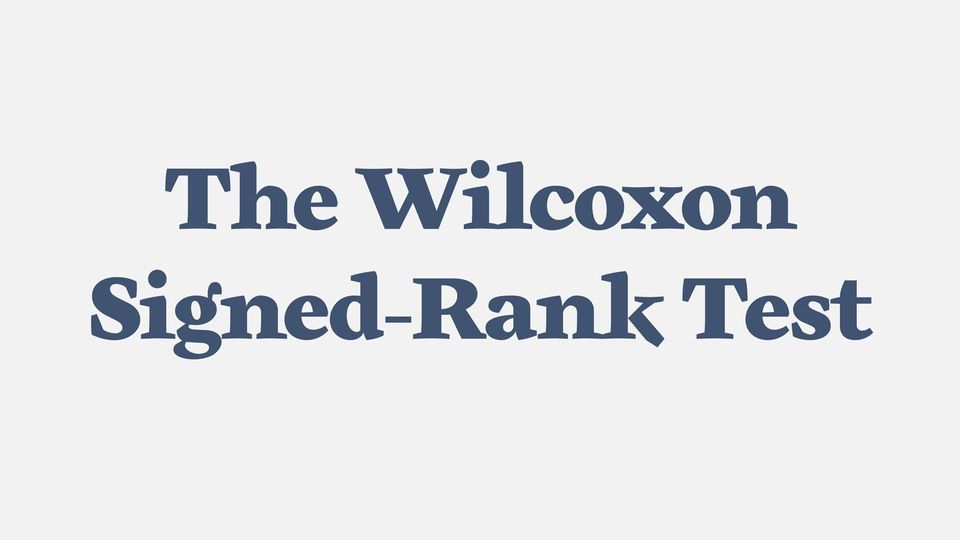
What is the Wilcoxon signed rank test?
The Wilcoxon signed rank test, which is also known as the Wilcoxon signed rank sum test and the Wilcoxon matched pairs test, is a non-parametric statistical test used to compare two dependent samples (in other words, two groups consisting of data points that are matched or paired). As with other non-parametric tests, this test assumes no specific distribution of the data being analyzed (for example, whether or not it takes a Normal distribution). The parametric equivalent to the Wilcoxon signed rank test is the dependent samples t-test (orpaired t-test).
The Wilcoxon signed-rank test can be used when comparing one sample to a specified value, as a non-parametric equivalent to a z-test or t-test. However, we focus here on the use of Wilcoxon signed rank test in the analysis of paired data. As with theMann-Whitney U Test和Kruskal Wallis测试, the Wilcoxon signed rank test is based on ranks assigned to the data points rather than the actual observed data.
The hypotheses for the Wilcoxon signed rank test for paired data are as follows:
- 零假设(H0)是人口中配对观测值之间的差异为零。
- The alternative hypothesis (H1) is that the difference between the paired observations is not equal to zero.
As with the Mann-Whitney U test, you can interpret this as comparing the medians of the differences between the paired observations but note that the medians are not actually involved in calculating the test statistic.
何时使用Wilcoxon签名的测试
As this is a non-parametric test of the magnitude of difference between paired data, it follows that the variable of interest should be continuous (able to take a number in a range) or discrete (data that can only take certain values). It is also important to understand what we mean when we say data are paired. Paired data arise when observations from one independent sample are uniquely matched or related to observations in another independent sample. This may be due to them coming from the same individual (such as duplicate measurements of blood pressure before and after a treatment) or from related individuals (such as a brother and sister or matched participants from a drug treatment vs control arm of a clinical trial). It is important that paired data are analyzed as such and not treated as independent samples.
非参数测试,也称为无分配测试,对数据的分布形状没有任何假设。当未达到数据正态性的假设时,它们用于检验假设。通常,这将是在不正常分布的小型数据集的背景下。
Wilcoxon签名的测试示例
在一项旨在评估新的缓解疼痛药物有效性的临床试验中,服用药物的疼痛水平为1至10,并将其评为1至10的疼痛水平。由于每个患者贡献了一个离散数字变量的一对数据点,因此样本量很小且不能假定正态性,Wilcoxon签名的对配对数据的级别测试适合测试对之间的差异。这可以手工完成四个步骤.
Step one: Present the null and alternative hypotheses
The hypotheses to be tested in this example are as follows:
零假设(H0)是测量前后测量之间的疼痛等级没有差异,或者对之间的中位差异为零。- The alternative hypothesis (H1) is that there is a difference in pain ratings between the before and after measurements, or that the median difference between the pairs is not equal to zero.
第二步:计算配对测量结果之间的差异并对其进行排名
为了找到数据对之间的差异,我们从治疗前的疼痛等级治疗后减去疼痛等级。测量值及其差异显示在表1中。请注意,当治疗后疼痛等级增加时,差异表示为负值。我们分配了忽略差异值是正还是负数的等级。
Patient ID |
疼痛等级(治疗前) |
Pain rating (after treatment) |
Difference |
Ranks |
1 |
8.0 |
6.5 |
1.5 |
2 |
2 |
6.0 |
5.0 |
1.0 |
1 |
3 |
3.5 |
5.5 |
-2.0 |
3 |
4 |
9.5 |
4.0 |
5.5 |
8 |
5 |
10.0 |
6.5 |
3.5 |
5 |
6 |
8.0 |
3.5 |
4.5 |
7 |
7 |
9.0 |
5.0 |
4.0 |
6 |
8 |
7.0 |
10.0 |
-3.0 |
4 |
表格1
Step three: Calculate the sums of the ranks to find the test statistic
Next, we use the ranks and calculate a sum of the ranks for the negative differences (W-) and a sum of the ranks for the positive differences (W+).
W-= 3 + 4 = 7
W+= 2 + 1 + 8 + 5 + 7 + 6 = 29
The Wilcoxon signed ranks test statistic is taken as the lowest of the two sums of the ranks, so in this case, our test statistic is W=7.
Step four: Obtain and interpret the p-value
Next, we determine a critical value of W with which to compare our calculated test statistic. We do this using areference tableof critical values. In this case, for our sample size of n=8, a significance level of 0.05 and a two-sided test (not specifying the direction of difference) our critical value is 3. Our calculated W statistic (W=7) is larger than the critical value, hence our p-value obtained is >0.05.
We can conclude that there is insufficient evidence to reject the null hypothesis in this example, and that there is no evidence to conclude a difference between the pain ratings before and after treatment.